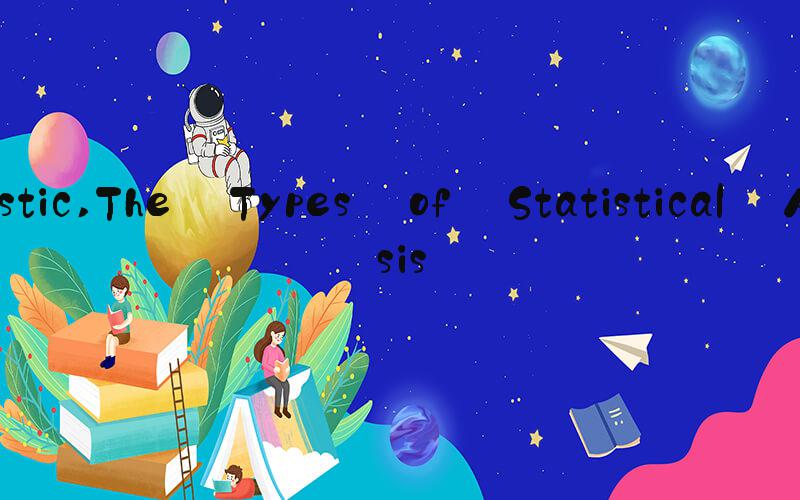
Statistical Analysis: An Overview
Statistical analysis is an essential tool in many fields like finance, healthcare, economics, and social sciences. In essence, statistical analysis aims to identify patterns, establish relationships, and predict trends based on data. In the era of big data, statistical analysis has become more important than ever, as industries rely on large datasets to make critical decisions.
The Types of Statistical Analysis
There are two primary types of statistical analysis: descriptive and inferential. Descriptive analysis involves summarizing and interpreting data, with the primary goal being to provide a summary of the key features in the data. On the other hand, inferential analysis aims to infer relationships and identify patterns between different groups of data, with the primary goal being to provide generalizations that can be applied to a broader population.
Common Statistical Analysis Techniques
Regression analysis is a type of inferential analysis that is used to determine the relationship between different variables. Typically the relationship between variables is determined by an equation, which helps calculate the probability of the relationship. Another common technique is hypothesis testing, which is a vital tool for inferential analysis. Hypothesis testing aims to determine whether a theory about a population is statistically significant based on a sample from that same population. Other common statistical techniques include cluster analysis, factor analysis, and standard deviation analysis.
Applications of Statistical Analysis
Statistical analysis has a broad range of applications across various industries. In the medical industry, for instance, statistical analysis plays a crucial role in identifying disease patterns, gauging the effectiveness of treatment programs, and predicting health outcomes. In finance, statistical analysis is crucial in predicting market trends, managing risk, and developing trading strategies. In social sciences, statistical analysis is useful in studying different populations, evaluating interventions and identifying trends.
Challenges in Statistical Analysis
While statistical analysis is a valuable tool, its application is not without its challenges. One of the significant challenges is collecting and managing big datasets. Large datasets require robust data management techniques and sophisticated analysis methods. Small sample sizes can also impact the accuracy of statistical analysis. In addition, statistical models can be complex and require a deep understanding of mathematical principles, making it difficult to interpret results for non-experts.
The Future of Statistical Analysis
The future of statistical analysis is tied to the continued growth of big data. As technology advances and data collection becomes more advanced, there will be a greater need for statistical analysis. New techniques and software will emerge to help manage big data more efficiently. Additionally, there will be a need for new statistical models that can handle the complexity of big data, including integrating artificial intelligence and machine learning. However, despite these advances, there will continue to be a need for skilled data analysts who can make sense of big data in real-time.
Conclusion
Statistical analysis remains a crucial tool for making sense of data in various industries. Its applications are broad, and as technology advances, data analyst skills will continue to be in high demand. The field of statistical analysis will continue to evolve as new techniques and software emerge to manage big data, making it possible to draw more conclusions from the sea of information we encounter in our increasingly digital world.